
See and Interpret the World with Scene Understanding

Computer Vision
-
New advances in computer vision have led to a revolution in image processing and are achieving superhuman-level performance on complex visual recognition tasks.
-
This exciting area of AI is evolving rapidly and is playing an increasing role in business, science, and technology.
-
Computer vision has wide applications across industries such as education, precision medicine, robotics, machine vision, automotive, precision agriculture, sports and entertainment, consumer and mobile, aerospace, conservation, and security.
-
It is used in an extraordinarily wide variety of problems and domains such as industrial inspection, drone, medical imaging, autonomous-driving, remote sensing, image retrieval, video surveillance, wildlife conservation, foundation models, generative AI, visual prompting, and more.
Real-World Applications of Computer Vision
Some Potential Applications
Medical – Medical practitioners are using computer vision for automatic analysis of 3-D radiological imagery.
Airport – Applications can count individuals in an airport to estimate how many people are entering the airport per hour to help the airport manage its operations.
Home Security – Smart doorbell systems can detect and recognize unknown faces and send notifications if a stranger turns up at the door.
Emotion Recognition – Manufacturers can register the emotional response of customers and potential customers to provide more innovative products based on user emotions.
Automotive – Automakers are embedding smart systems in vehicles that capture information regarding a driver's mental alertness in real-time to detect driver fatigue and improve safety.
​
Gesture Recognition – In the gaming industry, this new technology removes user interfaces for remote-controlled equipment and enables users to point to something or activate things without devices.
Quality Inspection – Farmers are using vision-based applications to manage product quality with an automatic visual inspection of crops and livestock.
Transportation – Tolling systems use optical character recognition to detect vehicle numbers from license plates for automatic toll collection.
Urban Planning – Smart cities use image segmentation to detect and count cars to manage traffic more effectively.
Energy – Utilities companies use computer vision to perform underwater visual inspections of structures and pipes to assist with predictive maintenance tasks.
​
Entertainment & Media – Various computer vision techniques are also being applied in filming, visual design, and post-production to enhance the creative and design process.
Remote Sensing – Significant work around applying computer vision to satellite imagery for building detection, road networks, land use classification, deforestation tracking, climate change, disaster recovery, wildlife conservation, resource management, precision agriculture and more.
Video Surveillance – Computer vision is assisting security and defense professionals with continuous video monitoring by detecting segments of video that contain suspicious activity in real-time and storing them for further analysis.
Autonomous Things – The automotive and aviation industries apply computer vision for autonomous entities (i.e. drones, self-driving cars, robots) that operate without human direction. Using data from images, sensors, radar, lidar, GPS, and maps, computer vision can assist with several tasks including aerial photography, obstacle detection, road detection, lane detection, pedestrian detection, brake light detection, speed limit sign detection, trajectory prediction, perception, motion planning, control and more.
Computer Vision: 3-D Medical Imaging Data Quality Control
Background
​
Medical errors cost the country billions of dollars and injure hundreds of thousands of people annually. Although several of these issues are difficult to solve without massive overhauls of business processes, most errors could be easily prevented using modern AI tools.
Solution
​
In this case study, we help Radiologists improve the error checking of a patient’s 3D body orientation in an image using machine learning. By applying state-of-the-art biomedical computer vision techniques, we predict a patient’s body orientation in CT scans. This means that incorrect metadata that was manually entered by a technician can be automatically identified and corrected to ensure patient records are as accurate as possible to improve diagnosis.

AI for Wildlife and Marine Conservation
-
New data acquisition technologies such as drones, remote sensing, motion-sensor cameras, and citizen science apps provide vast opportunities to help scientists analyze wildlife species and their ecosystems.
-
This enormous amount of data can play a significant role in wildlife research and conservation by automating image processing so that scientists can more easily detect, identify, and count wildlife in their natural environments.
-
AI can help address important problems including classifying images to understand the distribution of species, mapping resources and vegetation types, tracking the spread of diseases, predicting extreme weather events, assessing the impact of climate change, and protecting wildlife from poachers.
-
These smart applications will greatly assist conservation practitioners by automating time-consuming processes with faster, cheaper, and self-learning systems that enable them to focus on other challenging tasks.
Wildlife Image Tagging and Analysis
-
AI applications such as fine-grained image recognition can help scientists automatically emit different labels that are associated with millions of different species and then assigns image tags.
-
Previously, this manual task would rely on thousands of volunteers and hours to categorize photographs by species and behavior.
-
Researchers can analyze larger data sets more efficiently with computer vision to identify individual animals of endangered species while reducing fieldwork and image processing time.
Solution
In this case study, we process images from the Serengeti National Park and classify a zebra and the top categories identified in the photograph. Once an image is assigned with the correct tags, researchers can perform other advanced analyses to inform conservation policy.
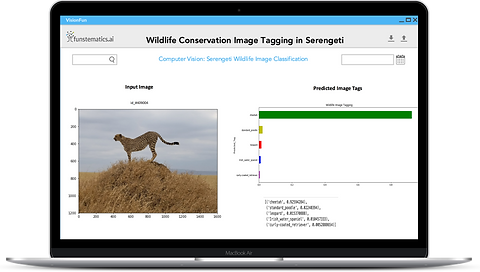
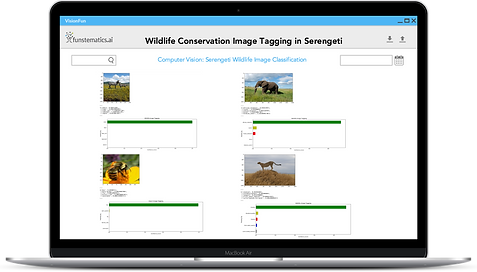
Object Detection – Remote Sensing Multispectral Satellite Imagery
-
Using modern cameras and advances in AI, new and unexplored territories of object detection applications can now be explored.
-
We can now define where things and people are in the real world, in real time and take action to improve safety and well-being.
-
Say you looked at a picture of different objects in a scene, you could immediately detect the classes and understand where in the image the objects are located using object detection.
-
Object detection could be applied to various business applications such as satellite imagery to study earth materials, detect hidden objects, and support natural hazard and disaster recovery.
Some of the ways we can sort through the remotely sensed data with AI to derive insights that people can use:
-
For instance, we might want to identify ports and understand shipping logistics to analyze economic trends and impacts on global trade.
-
In agriculture, we can identify what crop types are being grown in specific areas to better understand crop health and climate change impacts and optimize energy or water usage in those areas to maximize production.
-
During natural hazard and disaster events (earthquakes, wildfires, volcanoes, landslides, hurricanes, and floods), space imagery gives us a unique perspective of situational awareness to help deploy rescue teams and aid, and monitors things globally.
-
National security teams can analyze satellite imagery to identify undisclosed operating bases and construction activity.
-
Land use classification and detection with satellite imagery can provide socioeconomic indicators that help predict poverty and wealth in specific locations around the world.
-
AI can improve our ability to track developments such as roads, urban areas, waterways, farmland, and electricity to help organizations allocate funds and create more effective policies.
Solution
​
This case study demonstrates how complex vision tasks such as image tagging, segmentation, or object detection can be applied to identify aircraft in aerial or satellite imagery for surveillance of an airport. Additionally, we also demonstrate a satellite image land use and land cover multi-label classification system.
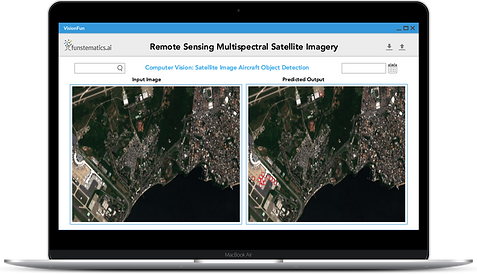

Image Segmentation for Scene Understanding
-
Rapid advances in computer vision are enabling brand new applications to be built that were impossible just a few years ago.
-
Image segmentation is a key component of image processing and computer vision applications such as autonomous driving, motion capture, and surveillance systems.
-
These segmentation models allow us to precisely classify every part of an image at an object instance level where the pixels of each individual object is located.
-
The applications of image segmentation at a pixel by pixel level are far-reaching and can be used to segment various types of complex objects and backgrounds for scene understanding.
-
Computer vision is now helping self-driving cars figure out where are the other cars and the pedestrians around it so it avoids them.
-
Image segmentation is also assisting medical practitioners with automatic analysis of 3-D radiological images.
-
Here we demonstrate how we use computer vision software to detect and segment objects such as humans, vehicles, road signs, wildlife, and also categorize each of these segments with a unique color.
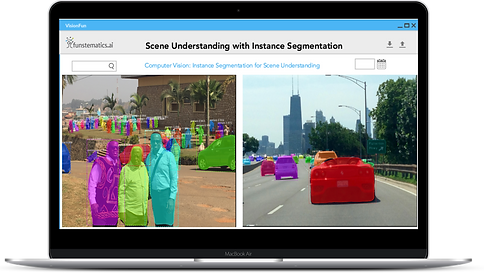
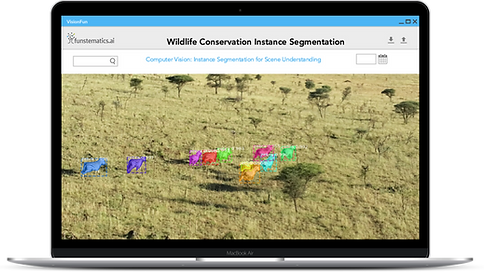
Sports Video Analysis and Tracking
-
With advances in modern communication and the internet, video has become one of the most common sources of visual information.
-
This vast amount of video data available online would take humans several years to watch all the videos available across various platforms.
-
Therefore, using automated tools for analyzing and understanding video content is essential to unlocking insights in video.
-
AI allows us to quickly and easily extract insights from videos and enhance content discovery experiences such as identifying objects of interest, detecting spoken words, and segmenting instances for more precisely.
Using computer vision and multimodal AI methods can improve video analysis across various tasks including:
​
-
Object detection and recognition
-
Instance segmentation and tracking
-
Scene understanding and crowd analysis
-
AR/VR highlight detection
-
Action recognition and event detection
-
Classification and captioning
-
Image Regression
-
Motion caption and classification
-
Event detection and recognition (motions, gestures)
-
People analysis (facial recognition, pose estimation)
-
Object tracking and segmentation behavior recognition
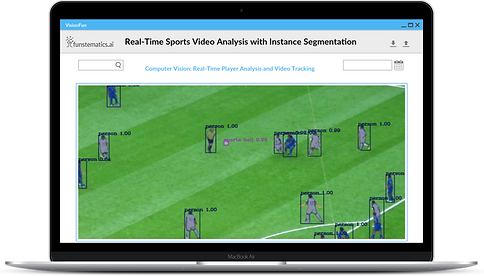


​
Fully Understand and Represent the Meaning of Natural Language
AI is a natural option for solving the numerous problems related to understanding human language. With the rich set of Computational Linguistics, NLP and ML tools available today, AI can help improve the performance of many hard NLP problems such as:
​
-
Parsing
-
Part of Speech Tagging
-
NLP Classification
-
Language Translation
-
Text Summarization
-
Name Entity Recognition (NER)
-
Sentiment analysis
-
Dynamic Topic modeling
-
Continuous Word Representations
-
Question and Answer
-
Prompt Engineering
-
Disambiguation
-
Image Captioning
-
Text to Image
Some of the most popular commercial NLP Applications include:
​
-
Spam detection
-
Legal document review
-
News articles categorization
-
Social media analysis and monitoring
-
Bio-medical
-
Security intelligence
-
Marketing and CRM
-
Sentiment analysis
-
Ad placements
-
Chatbots
-
Smart Speakers
-
Generative AI for expanding
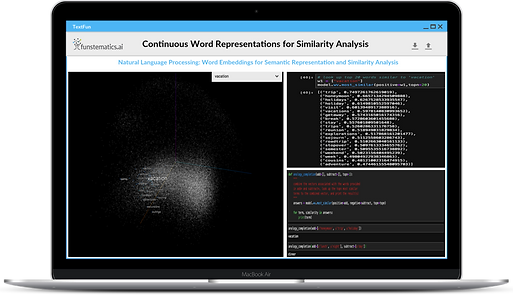
Customer Sentiment Classification Marketing and Retention
-
Creative use of advanced Natural Language Processing techniques can be an effective tool to develop desirable goods and services, anticipate customer desires, respond to customer problems, and stay ahead of competitors.
-
Using NLP, we can build applications that can extract key phrases to determine important points and topics, extract semantic meaning, and understand sentiment or intent from textual data.
-
With the recent advances in deep learning, the ability to analyze social media information, customer reviews on a website, email messages, or any message where it's important that we get a sense of the sentiment behind the text has improved considerably with human-level accuracy.
-
Techniques such as sentiment analysis and intent analysis are very important tools for analyzing textual data because it allows for segmentation by attitude, and could identify whether it relates to an opinion, news, marketing, complaint, suggestion, appreciation or query.
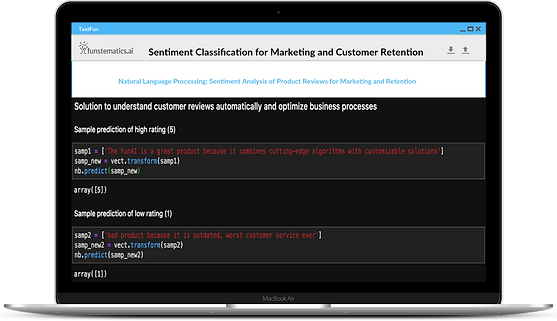
Intent Classification
Intent analysis steps up the game by analyzing the user’s intention behind a message and identifying whether it relates an opinion, news, marketing, complaint, suggestion, appreciation or query.

-
Our society is rapidly evolving with respect to innovative technology, trade, business, and media.
-
We now have the internet and various forms of communication platforms that we consume to conduct business, stay aware of daily events, connect with the world, and communicate with our loved ones.
-
These advancements are generating huge amounts of text and unstructured data that need to be quickly analyzed to extract valuable insights.
-
This produces a significant challenge in business because the presence of excess data or information becomes difficult to process in making well-informed decisions, and humans have short attention spans when consuming large text documents and articles.
-
However, AI can be applied to perform automated text summarization to shorten huge documents into a summary that captures the key concepts and meaning of the original document for consumers and clients.
-
Some common approaches for building automated text summarizers and information extraction systems include extraction and abstraction-based techniques such as natural language generation (NLG), ranking models, topic models, and key phrase extraction.
-
The core concept behind all these applications is to find a representative subset of the original dataset that retains the semantic and conceptual points to generate actionable insights.
Document Summarization
-
In this case study will demonstrate a simple implementation of document summarization to solve the challenge of information overload.
​
-
We will show from the outputs that we successfully summarized our document into a shorter summary that depicts the core essence of the document, and also supports keyword extraction.

Machine Translation with Attention
-
One of the most significant language processing applications that plays a central role in our multilingual information society is neural machine translation.
-
The goal of this model is to build an end-to-end network that can read in a sentence then output a translation directly.
-
This technique can help with providing syntactic, grammatical, and semantically correct translation between any two pairs of languages such as English to French.
-
Modern sequence-to-sequence models use encoder and decoder architecture to encode a source sentence into a fixed-length vector from which a decoder generates the respective translation.
-
These AI systems provide enormous social, government, and commercial value by providing more natural human-machine translation interfaces, and access to valuable stored information.
-
More significantly, this is a very powerful way to bring people together from different languages, different countries, and enable them to improve communication and collaboration.
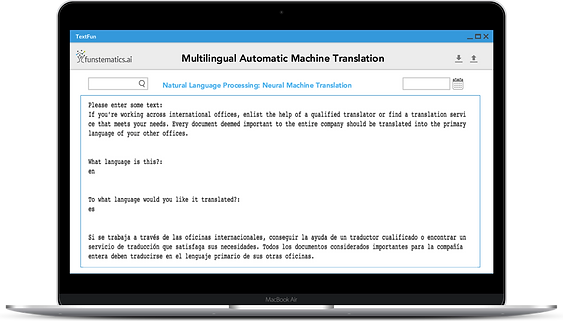
Neural Machine Translation Workflow
-
Input Text
-
Encoder-Decoder
-
Translated Text
In this case study, we demonstrate how we can use neural machine translation to submit some input text in one language and have it instantly translated to another language of our choice.
-
With the wealth of AI capabilities that are now rapidly becoming available, businesses are engaging their customers in new transformative ways with conversational agents called chatbots.
-
Using state-of-the-art machine learning, chatbots can hold contextual conversations with users, perceive vast amounts of information, and respond intelligently using speech or text.
-
These AI agents are being used in customer service, advertising, and marketing to deliver more customized experiences.
-
Chatbots can also act as a personal assistant to help answer your questions, automate your home, book reservations, order food, or purchase an item.
-
Most importantly, chatbots can support and scale business operations, increase customer satisfaction, boost brand value, save time and money, automate repetitive tasks, and become personal assistants.

Speech Recognition & Synthesis
-
Speaking and writing are two of the most common forms of human communication in our everyday life products such as telephones, computers, radio and television, video games, audio players, and mobile devices.
-
As a result, vast amounts of data important to several industry domains are being stored in the form of written documents and recorded speech.
-
The ability to convert this spoken and handwritten data into digital texts or audio can lead people to interact more naturally with computers.
-
With recent advances in automatic speech recognition and foundation models, computers can now understand speech and text, create dialogues with humans in natural ways, and complete actions and tasks on behalf of users through automatic speech recognition and speech synthesis.
-
Automatic speech recognition refers to the task of transcribing audio into text, while speech synthesizers generate artificial speech by converting normal language text into audio.
-
Increasingly, AI systems enable humans to communicate with software and hardware services through speech recognition.
-
These techniques are enabling successful applications and automation in customer care systems, smart speakers, speech translation, spoken document retrieval, voice recognition, performing authentication, assisting the disabled, and conversational AI.
-
Speech recognition applications are also transforming various industries by reducing business costs, automating complex processes, and increasing overall efficiency.
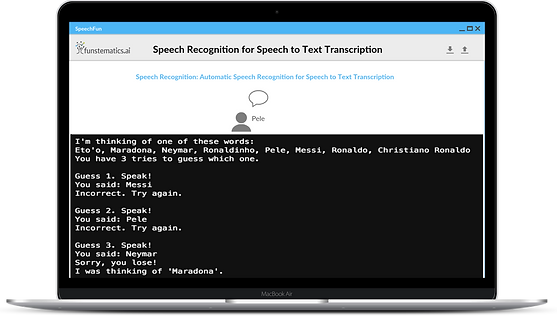
Conversational AI with Speech Recognition & Natural Language Understanding (NLU)
-
Increasingly, we expect chatbots and smart machines to be able to use AI in order to understand spoken or typed commands in natural language and speech.
-
To make this possible, we need to apply speech-to-text transcription to generate text from user commands and text-to-speech to convert the system responses back into audio.
-
Now to work with speech, we have to recognize that there are two models that need to work together.
-
First of all, there's an acoustic model that matches audio to sound units or phonemes, which define how specific word fragments are pronounced in a given language.
-
Then there's a language model that provides a probability distribution across a sequence of words.
-
In order for computers to be able to interpret user input, modern AI systems combine speech and language understanding technologies such as domain classification, intent classification, and semantic slot filling to enable humans to communicate with software services.
AI-powered Home Automation Using Speech Recognition and Natural Language Understanding
In this use case, we use intelligent voice-activated control to say ”Turn on the light" or ”Put the light on", and have an AI-powered device understand the spoken command and take appropriate action.

Natural Language Processing
-
As humans, having the ability to communicate naturally through written and spoken language is key to successful collaboration.
-
Natural Language Processing (NLP) allows us to build deep learning algorithms to solve critical tasks that can process text and speech to enable AI for language and communication. For example, NLP applications can help us understand text, extract semantic representations, embed neural knowledge graphs, determine important points and topics, analyze sentiment, generate text, and even speak and engage in conversations with AI agents.
-
Our modern NLP methods will enable you to automatically search, retrieve, prompt, and analyze textual data using efficient pre-processing tools, computational linguistics, topic models, embeddings for similarity analysis, text categorization, text visualization, transformers, foundation models, and deep learning models.
-
We use highly sophisticated multimodal vision and language joint learning and inference applications including natural language understanding, machine translation, semantics and the syntactic parsing, continuous word representation, automatic summarization, natural language emulation, dialectal systems, image captioning and visual question answering, information retrieval, semantic parsing, dialect systems, entity ranking, Chatbots, knowledge graphs, and deep reinforcement relevance networks.
-
Our NLP workflow will guide you through the process of analyzing your raw textual data using a combination of efficient preprocessing tools, computational linguistics, topic models, distributional semantic word representations, semantic similarity analysis, text classification, text generation, visualizations, prompt engineering, transformer architecture, large language models (LLMs), and NLP deep learning models.
Continuous Word Representations
-
Traditional methods like one-hot encoding can be considered as a kind of high dimensional embedding but they provide no useful information to the natural language processing (NLP) systems regarding the relationships that may exist between the individual vectors.
-
In the case of word embeddings, it can benefit a lot of different NLP tasks by mapping words or phrases to vectors of real numbers to a lower dimensional space, then assign the semantic meaning to this representation.
-
This new lower dimensional vector space also places words that are semantically similar or relevant to each other close to each other in this semantic space.
-
Additionally, word embeddings can be used for a variety of text processing tasks such as feature extraction and language modeling.
Continuous word representations are useful for several NLP problems such as preprocessing, semantic representations, text classification, recommendation systems, machine translation, spoken dialogue systems, synonyms and search query expansion, question answering, and more.
In this task, we assign the semantic meaning to this representation and compute the semantic representation.
​
Some continuous word representations include:
​
WordRank, FastText, Multi-Relational LSA, RDA, CBOW/Skip-gram, RNN-LM, SENNA, DSSM, GloVe, Word2Vec, Doc2Vec, Item2Vec, Brown Clustering, VarEmbed etc.
​
Other Techniques:
​
-
Word Algebra, t-SNE
-
Automatic Phrase Modeling
Topic Modeling
-
As more massive collections of electronic text become available to us, automatically extracting valuable information from this unstructured data is incredibly valuable for consumers.
-
To maximize this hidden value, software needs to be able to create structure, summarize text, extract relationships and semantic meaning, and even sentiment information from the language we use as humans.
-
Topic Modeling is an information retrieval and extraction technique to systematically organize, understand, and discover topics in large collections of text documents.
-
It can help us discover document-level themes in large textual data, annotate documents with the learned topics, and support a variety of subsequent NLP tasks including organizing, searching, understanding, visualizing, predicting, and summarizing unstructured information.
-
This suite of tools can be used to help explore, summarize, and perform predictions across many domains including images, music, networks, genomics, and neuroscience.
​
Some applications:
Topic correlation, Document clustering, Document deduplication, Document analysis, Dynamic Topic modeling, Author Topic modeling, Collaborative Topic modeling, and Topic Networks etc.
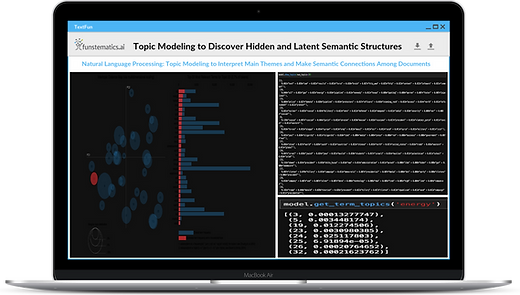
Sentiment Analysis
​
Suppose you want to build an NLP system to examine millions of online product reviews and automatically tell you if the customer liked or disliked a specific product or service.
For example, you hope to classify the following review as highly positive:
"This is a great elliptical machine and I use it every day!"
And the following as highly negative:
"This bike is low quality--I regret buying it."
The problem of recognizing positive vs. negative opinions is “sentiment classification.”
​
Sentiment classification is a technique that we can use to analyze text and try to discern whether the text indicates that the writer is happy or unhappy or perhaps neutral about something.
Automatic Text Summarization
Natural Language Understanding
-
Natural Language Understanding (NLU) is a task to interpret conversational speech or text to perform a series of tasks based on the user's request.
-
Designed to identify valuable information in conversations, language understanding uses machine learning to detect user intents and entities from sentences to integrate natural language understanding into apps, bots, and IoT devices.
-
Using advanced dialogue management based on machine learning, you can build smart bots and digital assistants that can handle multi-turn, contextual conversations like Siri, Cortana, or Google Assistant.
​
​
Applications:
Domain Classification, Intent Recognition, Semantic Slot Filling.

AI Bots and Digital Assistants for your industry
Customer Service Chatbot (NLU)
-
AI bots are capable of human-level speech and comprehension.
-
They can communicate with humans, understand needs, and help with tasks that boost our quality of life and global productivity.
​
​
Embed AI bots into web applications and mobile devices.
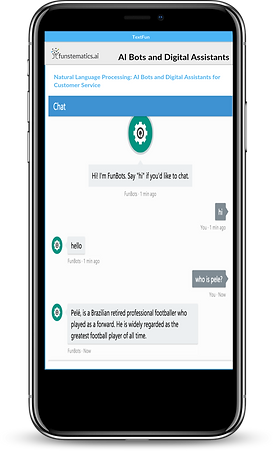
Image Captioning
-
Humans learn to interact with the world using a combination of natural language, images, sequences, and knowledge jointly.
-
This multimodal learning helps us acquire information from diverse sources and learn to be smart and perform intelligent tasks.
-
So for building machine intelligence, we also hope the machine can learn from multiple sources and perform intelligent tasks across multiple modalities.
-
One example of multimodal learning is image captioning, where an AI system generates a caption for an image.
-
Another example is phase to image, where NLP and Computer Vision techniques can be combined to go from a sentence to an image.
-
By building machine intelligence to understand images deeper, we can describe objects, attributes, and relationships in a more natural way to communicate with humans.
​
​
​
Several benefits can be obtained by people who would benefit from automated insights from photos or videos.
​
Some examples include:
​
-
Automatically generating text and extracting insights from a broad range of visual concepts.
-
Serving as an assistance tool for handicapped and visually impaired individuals.
-
Explaining and understanding what happens in a video in real-time.
-
Supporting a variety of autonomous devices and applications.
MSR Image Captioning Workflow
-
Image Word Detection
-
Language Model Generates Caption Candidates
-
DMSM Caption Re-ranking

Speech to Text Transcription
In this case study, we demonstrate how automatic speech recognition can be applied to develop a simple speech processing program to transcribe speech into text.
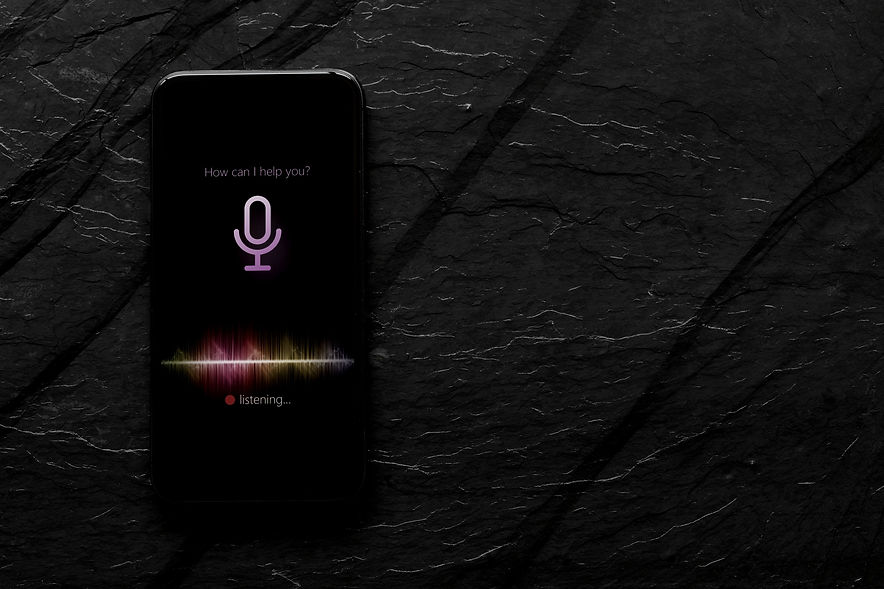
Build Smart Conversational AI Products
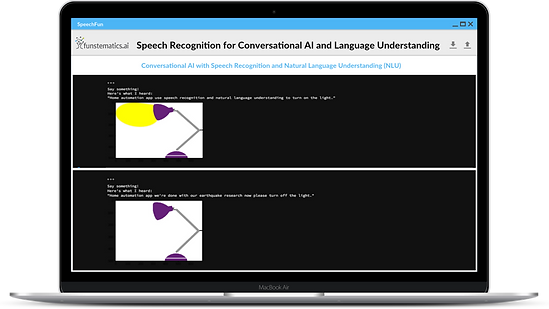

Automatically Learn to Maximize Rewards
Agent-Environment Interface

Backup Diagram

Reinforcement learning agents learn to interact with dynamic environments and attempt to optimize their actions to maximize the rewards received.
Deep RL is allowing machines to achieve superhuman performance in 2D or 3D simulated worlds, video games, and board games such as Atari, Pac-Man, Go and more.
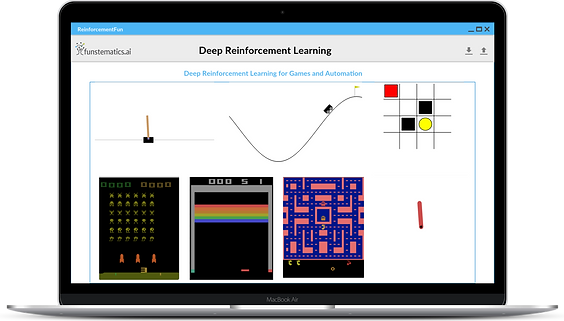
-
We are really excited about how far reinforcement learning has come in areas such as gaming and robotics, but we are most passionate about is how far it can go in other real-world applications.
-
Having agents that can be transplanted from a complicated game such as Go to any other domain should mean now we can tackle some of the most challenging and impactful problems facing humanity.
-
Untie yourself from the specifics of the domain you are in and you come up with an algorithm that is so general that it can be applied anywhere.

Deep Reinforcement Learning
-
Some of the most notable advances in AI have involved reinforcement learning with function approximation by deep neural networks.
-
Deep reinforcement learning is an enormously exciting area of AI because many practical applications can be modeled as reinforcement learning problems.
-
With its focus on learning by interacting with dynamic environments, deep RL provides a framework for representation learning where an objective can be achieved with minimal domain knowledge.
-
Embedding reinforcement learning agents into applications can also amplify and extend human abilities by considering long-term consequences.
-
Reinforcement learning has been successful in a wave of real-world applications of AI such as aiding human decision making, playing games, robotics, exploring simulated worlds, and improving customer experience.
Reinforcement learning’s ability to automatically learn to behave to maximize rewards can boost productivity across many fields:
-
The gaming industry uses reinforcement learning algorithms to play traditional board games and video games such as Go and Atari, achieving superhuman level performance.
-
Manufacturing factory robots use reinforcement learning to assemble and create various products.
-
Decision support systems use reinforcement learning in areas such as education, transportation, healthcare, energy, and public-sector resource allocation.
-
Inventory management applications use reinforcement learning to reduce transit time in stocking and utilize space more efficiently.
-
The automotive industry uses reinforcement learning for multimodal tasks such as object recognition, planning, routing, and object avoidance.
-
Delivery management systems use reinforcement learning to solve the problem of vehicle routing optimization.
-
Financial services apply reinforcement learning for accounting and algorithmic trading strategies.
RL techniques:
Contextual Bandit, DP, MC, TD, DQN, Policy Gradients, Actor Critic, REINFORCE, AC3, Deep RL
This case study will demonstrate how we apply reinforcement learning to learn what to do by mapping situations to actions in order to maximize a given reward.
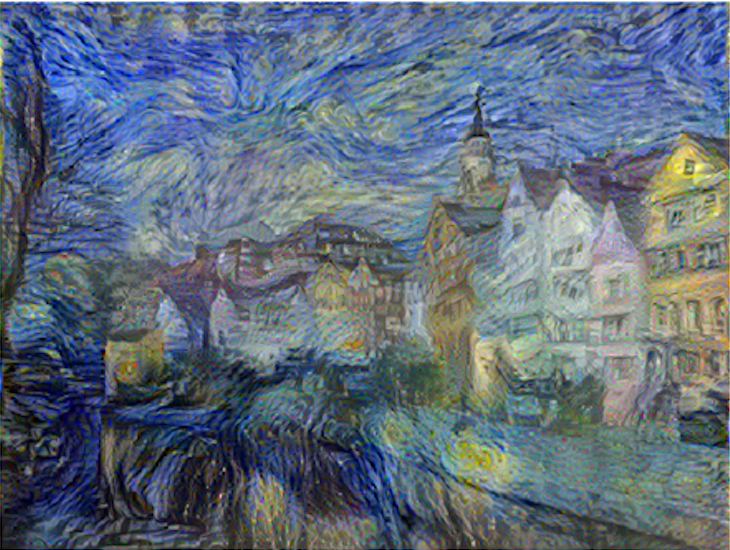
Computational Creativity
Generative AI and Computational Creativity
Apply generative models to:
-
Improve models by simulating training data
-
Imputation on missing data
-
Simulate the world as “it could be” rather than as “it is presented.”
-
Generate realistic images, video, audio, text, and art
-
3D generation
-
​Unify tasks with prompt engineering
-
One-shot learning
-
Control representation learning
-
Do simulation by prediction
-
Solve difficult prediction problems
-
Learn useful embedding representations
GANs
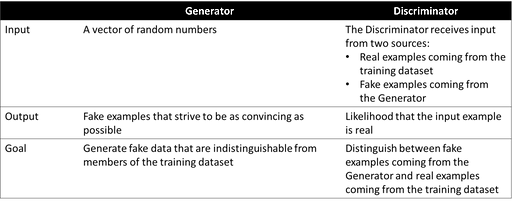

Using Generative Modeling To Make New Things
-
The potential of AI as a tool to augment human capabilities goes well beyond annotating existing data and into generative and creative activities.
-
A new wave of creative applications of AI is making it possible to automatically generate text, audio, speech, and images.
-
These generative models offer some really exciting opportunities in AI because they augment human creativity and are incredibly important in the context of data reproduction without human supervision.
-
The motivations for generative modeling include density estimation or realistic sample generation tasks.


-
The idea of generative models is to be able to learn the probability distribution of the training set and create more data from the original data.
-
An exciting technique Generative Adversarial Networks, which consist of two models competing against each other, the Generator and Discriminator, the optimization process seeks an equilibrium between two forces.
-
The Generator network replicates real data from fake data, and The Discriminator network distinguishes real data from fake data.
-
Discriminator loss is passed to Generator as an objective function
-
Adversarial frameworks and transformers are very powerful and solve a lot of different tasks including image and video generation, domain translation, image super resolution, colorization of black and white photos, anomaly detection, drug discovery, art generation, image inpainting, speech synthesis, and semi-supervised learning.
​​
​
Generative Techniques:
​
VAE, DCGAN, WGAN, CGAN, CyleGAN, LLMs, Foundation Models, and more.

Model Complex Network Relational Problems
Network Science and AI
-
Reasoning about entities and their relations in linked data is a key problem in network analysis and AI.
-
Graph theory and network science have had a huge impact across several domains by enabling people to analyze complex graph-structured representations of knowledge.
-
Many of the top internet companies are creating significant value from building and analyzing dynamic networks of relationships to outperform competitors and create new innovations.
-
Network science and AI are key components for many of today’s highest impact applications such as web search engines, online social networks, information networks, product recommendations, communication networks, and transportation systems.
-
These techniques can be applied to problems in nearly any domain including healthcare, politics, science, law, education, and finance to answer questions about how network systems work, and how entities behave within it.
-
By characterizing the structure of these network representations, we can apply AI to better understand how structure ultimately influences performance to maximize business success.
Airport Transportation Network
Using network science to model and optimize US airport transportation networks.
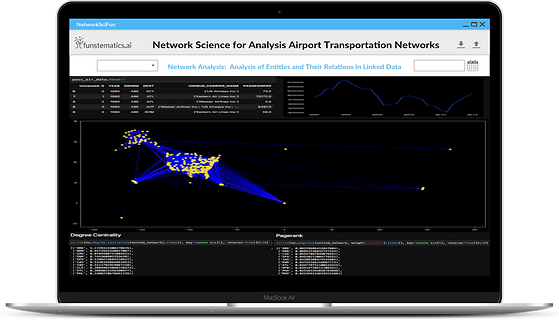
Network Graphs
Mathematical structure used to model pairwise relations between objects, where the objects are usually called nodes and the relationship between them edges.
G = (V, E), directed/undirected
V = set of nodes/vertices
E = set of (x, y) edges
Applications:
​
-
Understand centrality measures
-
Track evolution of importance of entities over time
-
Analyze connected components
-
Compute shortest paths in a network
-
Small World (Six degrees of separation, Erdos Number, Bacon Number etc.)
-
Clustering, Community Detection, Sub-networks
-
Network Performance Optimization and Prediction
-
Graph Neural Networks (GNNs)
-
Knowledge Graphs

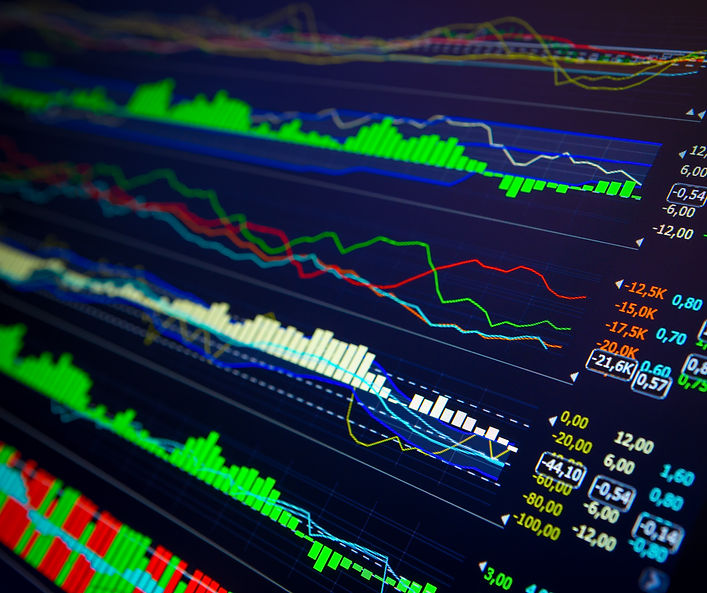
Solve Interesting Tasks Where the Target is a Set of Continuous Values

IoT Solar Energy Forecasting
-
Some of the most interesting problems for enterprises today require mapping a sequence either as an input or an output.
-
Many of these sequence input or output mapping problems are being solved with state-of-the-art AI algorithms and foundation models.
-
Tasks that involve sequence representations include time series forecasting, sentiment analysis, multimodal image captioning, spoken language understanding, neural machine translation, and continuous video classification.
-
Support for sequence data is an important class of problems across several industries, and one where AI is producing remarkable results that are boosting productivity for many enterprises.
-
AI is being used to revolutionize the energy industry in various areas including energy forecasting, distribution, smart metering, energy efficiency, and predictive asset maintenance.
-
One industry that is being revolutionized by AI is the energy sector with important applications in energy forecasting, distribution, smart metering, energy efficiency, and predictive asset maintenance.
-
While AI can be applied to a variety of sequence problems, we will demonstrate how deep architectures can be used for energy forecasting to manage excess demand, prevent disruptions, and reduce waste.
-
Predicting solar energy usage in IoT data is an essential task for efficient management and utilization of resources because every marginal improvement can have a huge impact.
IoT Solar Energy DL-based Time Series Forecasting
In this case study, we demonstrate how DL-based time series architectures can be used to predict the daily total power production of a solar panel for a future day based on the initial readings of the day.
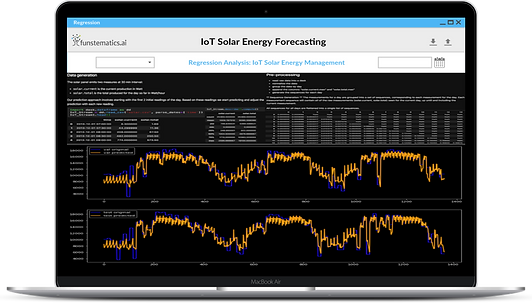

Learn Abnormal Patterns With or Without Supervision
Applying Kernel Methods to Identify Abnormal Transactions
Anomaly Detection Approaches:
​
-
Classification & Regression
-
Clustering
-
Kernel
-
Density
-
Nearest-neighbor
-
Generative
-
Statistical
-
Subspace
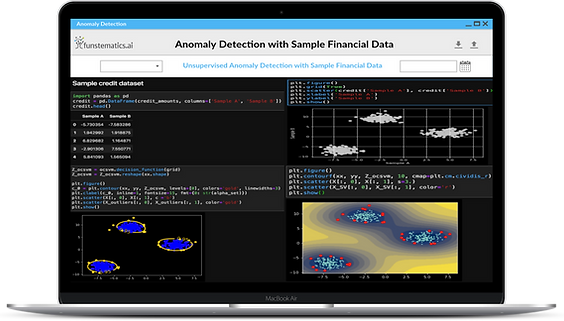

AI for Anomaly Detection
-
Anomaly detection is a vast and very complex problem that deals with finding unusual things and outliers in a dataset.
-
Finding new types of anomalies that have never been seen before in an ever-changing and more sophisticated environment is a critical task that enterprises have to tackle in order to detect fraud, network intrusions, machine equipment failures, and server problems.
-
AI techniques are very useful for these tasks because they can automatically learn abnormal patterns in data in a supervised or unsupervised way.
-
These methods are already proving capable of solving some of the most challenging anomaly detection problems that enterprises struggle with such as credit card fraud, cyberattacks, medical diagnosis, anti-money laundering, manufacturing quality assurance, and data center monitoring.
-
Typical AI approaches well suited for anomaly detection include classification and regression models, exploratory data analysis, unsupervised novelty detection, generative models, and network analysis.
Unsupervised Anomaly Detection with Sample Financial Data

Solve Interesting Problems with Multi-label Categorical Target Variables

Credit Risk Loan Default Classification
Credit Risk Modeling for Loan Default Classification
-
Modeling credit risk is of major importance for financial institutions who offer loan products and face the risk that a customer may default on a debt by failing to make the required payments.
-
Having the ability to analyze the probability that a debtor will default is a critical component for measuring credit risk.
-
This problem can be easily managed by building an intelligent loan approval pre-check system for potential customers using personal data collected during the loan application process.
-
With recent advances in AI and foundation models, risk can be significantly reduced by finding new ways to use customer data for credit analysis and being able to predict the outcome of these loans.
AI provides tremendous benefits for consumers and lenders such as:
-
Automated credit scoring process
-
Increased profitability
-
Faster loan process
-
Healthy lending market
-
Regulatory compliance
Typical Workflow:
-
Identify relevant data set(s)
-
Preprocess the data set
-
Exploratory Data Analysis
-
Train the model
-
Check the performance of the model
-
Tune the model
-
Deploy the model
-
Iterate and collect more data to enable retraining
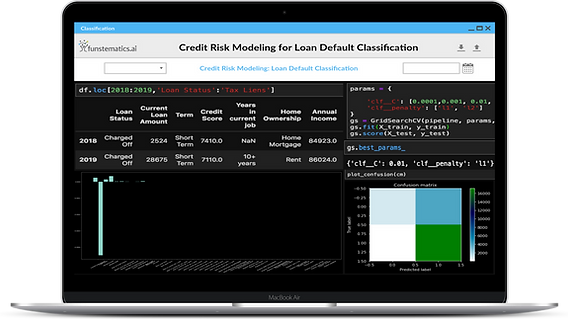
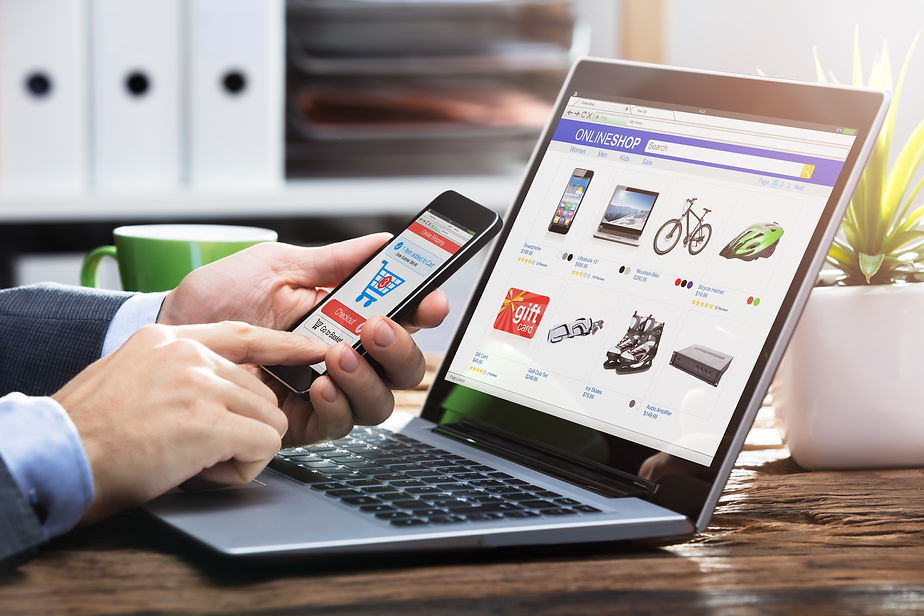
Automatically Learn Ratings and Preferences of Users

AI Recommender Systems for E-commerce
-
Most of today’s largest e-commerce and social networking platforms use recommendation systems to generate value by improving user experience, cross-selling or up-selling products, recommending related connections, social networks, and digital marketing.
-
The goal of a recommender system is to identify and suggest items that are most relevant to a user such as products and connections.
-
Some common approaches to recommendation systems include, transactional-based collaborative filters (CFs), Content-based CFs, Collaborative Filtering with Embeddings, Deep learning RS Algorithms, and Hybrid methods.
-
These recommendation applications may use data from historical transactions, user ratings, user behavior monitoring, user information, item details, user demographics, social networks, location, time, and other IoT systems.
-
Recommendation systems create a ranked list of relevant items for the user taking into consideration a specific context such as products, services, jobs, music, friends, groups, food and more.
Music Recommender System
Music Recommender with Personalization - Item Similarity Based Collaborative Filtering
In this case study, we will demonstrate how a sample recommender system can be used to suggest songs to a user using collaborative filtering.
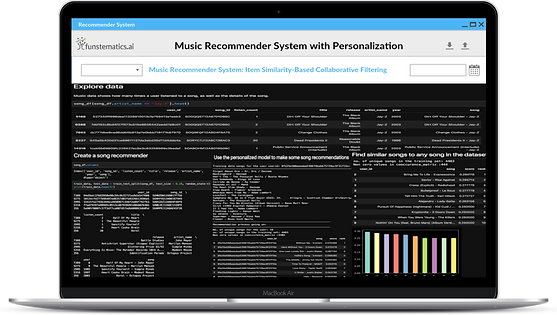
Have a new product idea, or need help spotting feasible and valuable AI projects for your business? Partner with us to get started with a pilot project.